10 Recommendations for Fab Cycle Time Improvement
Concrete tips for making operational changes in the fab to drive cycle time improvement and improve profitability.
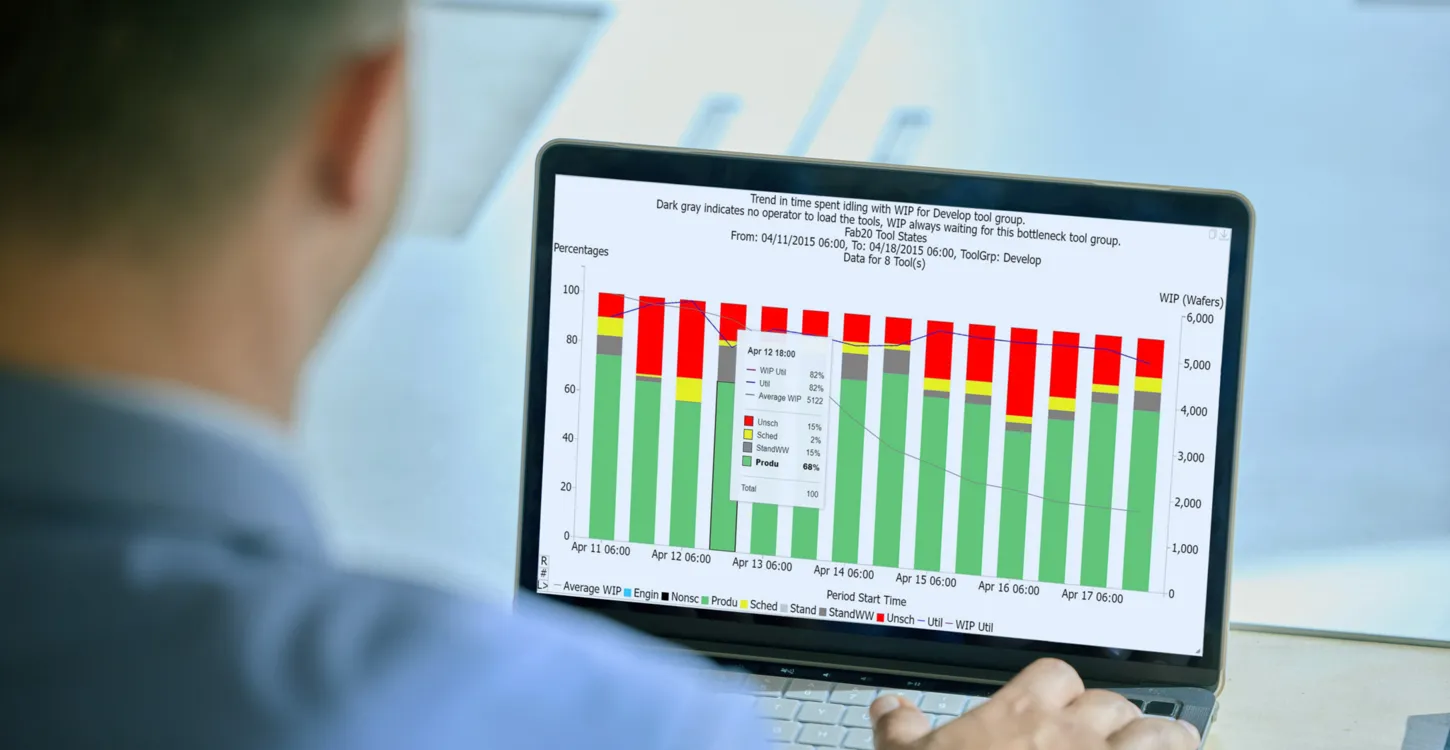
Background: Cycle time increases during times of high demand
Readers of the FabTime newsletter understand well the core relationship between cycle time and capacity utilization in a fab. In the presence of any variability (which we will always have in fabs), cycle time increases rapidly as a fab approaches 100% utilization of the bottleneck(s). At times when the industry faces demand pressure, fab cycle times can surge in many sectors.
While demand-driven cycle time problems can be mitigated by capacity expansion (either by building new fabs or by adding equipment to existing fabs), this is not a quick process. Fabs are expensive and complex and can take years to build from scratch. Thus, efforts to improve fab cycle time by making operational changes are of particular value during times of high demand. These types of improvements, which center on reducing variability to move to a more favorable operating curve, are at the heart of our educational outreach via the FabTime cycle time management course and newsletters.
For many years, we concluded our course with a slide that lists our top ten recommendations for improving fab cycle time. These recommendations, sorted in “bang for the buck” order, recap topics discussed in more detail throughout the course.
1. Identify and eliminate single path operations (if possible)
As discussed in Issue 20.05: The Impact of Tool Qualification on Cycle Time, one of the fundamental drivers of cycle time at the tool group level is number of qualified tools. This is because, in the presence of variability, having another potential path to be processed significantly reduces the chance that a lot will have to wait. When there is only a single path for an operation, any variability at that tool impacts all lots in queue, whether this variability is in the form of unscheduled downtime, scheduled downtime, engineering time, operator delays, or long process times of other lots. Having a second path, at the same utilization level, decreases the average cycle time through an operation by approximately 50%. Adding a third path decreases the cycle time by another 20-30%, with the relative improvement tapering off after that.
This behavior is illustrated by the graph below, which shows varying levels of cross-qualification for 10 operations and 10 tools. On the left is full dedication (one tool per operation). On the right is full cross-qualification (any of the 10 tools can run any of the 10 operations).
Sometimes, of course, a fab will have true one-of-a-kind tools. In this case, there is not very much to be done about those single paths, except to prioritize such tools for any capacity expansion projects and work in the meantime to minimize variability on those tools.) See Issue 23.05: Managing One-of-a-Kind Tools for other ideas.)
However, it is often the case that tool qualification choices lead to single path operations. Efforts to qualify a second tool are of high value, reducing average cycle time through the operation by about 50%. This is a low cost, high benefit solution. The FabTime team's number one recommendation for improving cycle time without adding capacity is to identify single path operations and work to qualify at least one more tool for each.
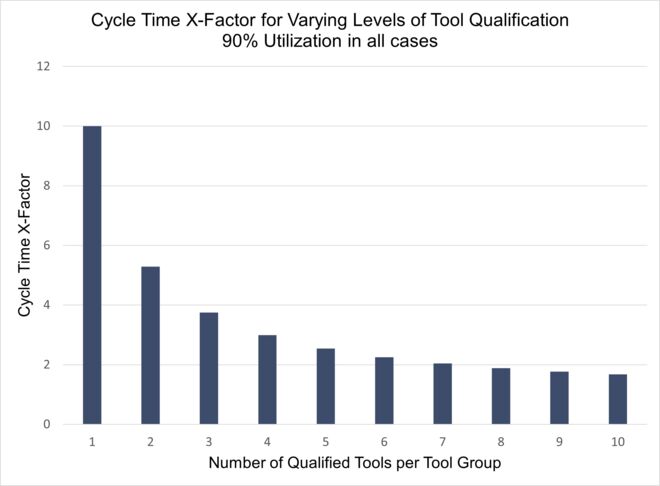
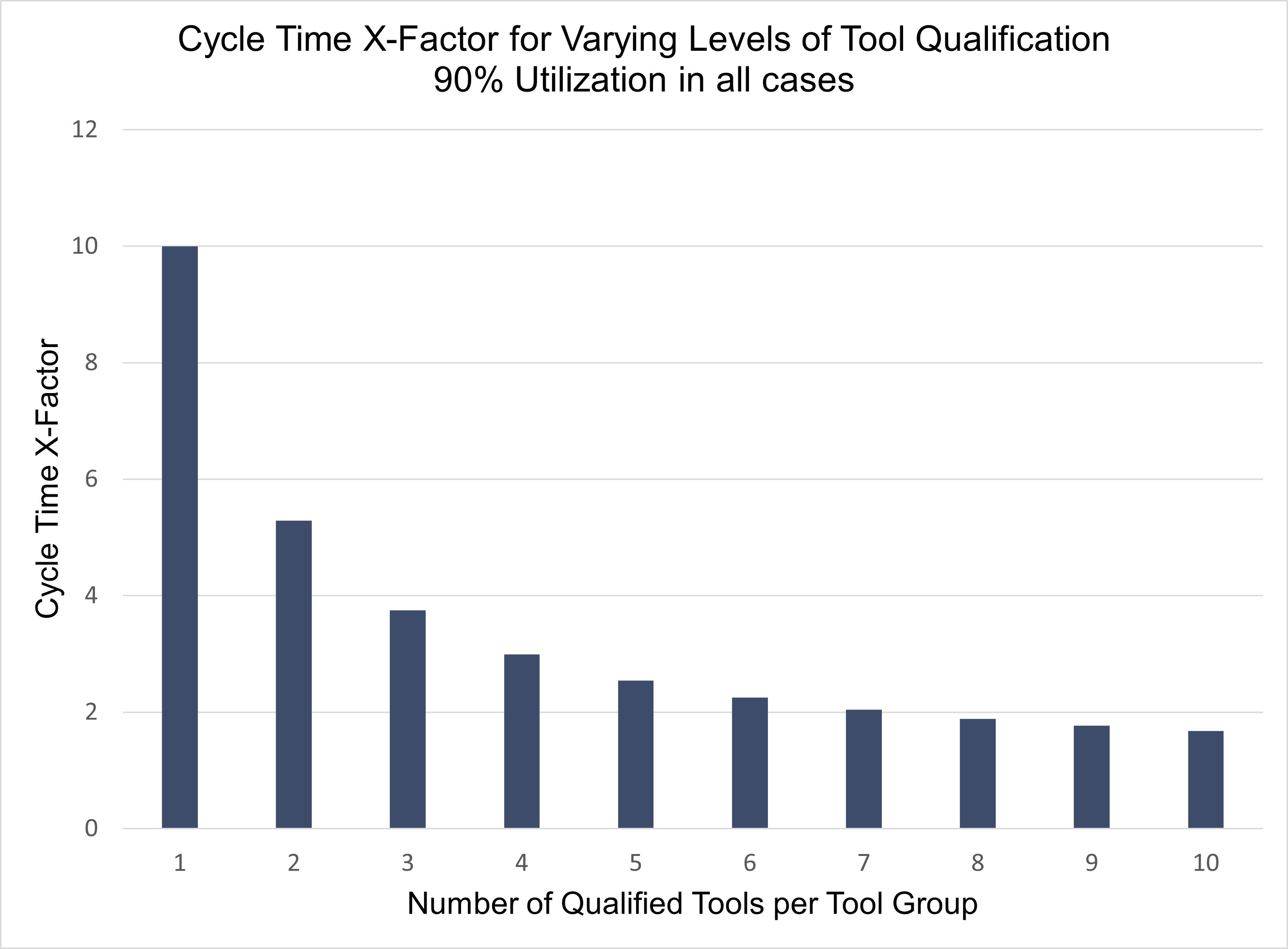
2. Check for soft dedication due to operator preferences
Sometimes, even when engineers qualify multiple tools for every operation, a closer look at the data reveals “soft dedication”. This is when multiple tools are qualified to run a particular operation, but the operators for some reason prefer one tool over the other(s). This can happen because of:
- Layout issues (the other qualified tools require more travel time)
- Tool capability differences (one tool is faster, or easier to use)
- Data communication issues (not everyone knows that another tool has been qualified)
What can happen in this case is a load imbalance across the tools. One tool ends up being operated at a higher utilization than necessary, while another has excess capacity. Because cycle time increases non-linearly with utilization (especially as you get closer to 100% utilization), this leads to increases in overall cycle time. It’s a hidden source of cycle time.
To identify soft dedication, start by looking at the moves relative to availability efficiency by tool for key tool groups or capacity types. If a tool group is fully cross-qualified, you can expect this to be similar across tools. Where one tool seems to be used more (or less) than the others relative to availability, there may be soft dedication occurring. An unexpected difference may be cause for further investigation.
It’s important to add that just because the operators are doing something that isn’t planned for in your capacity model doesn’t mean that they are wrong. The reason for the soft dedication may be a good one. We once worked with a fab where we observed that one tool had a consistently lower than expected number of moves, after adjusting for availability. When we asked about it, our contact said “Well, that tool is actually in a separate building from the other tools.” We said something along the lines of “Aha!”. In that example, we recommended modifying the capacity planning model to reflect the soft dedication that was occurring in practice.
3. Reduce transfer batch sizes between steps
In less automated fabs, transfer batching can be another hidden source of cycle time problems. Where carts are used to transfer lots between steps, operators have an understandable bias towards efficiency. They often wait until a cart is full before transferring any of the lots to the next step. While this is efficient in terms of reducing steps for the operators, it is terrible for cycle time. Queue time is introduced as the lots wait upstream, especially for the first lot added to the cart.
Then, when the lots are unloaded, a bubble of WIP arrives all at once to the next step. This arrival variability introduces queue time downstream. We have worked with fabs that reduced the overall cycle time of a fab by purchasing smaller carts, or by hiring dedicated runners to transport lots. These solutions aren’t free, but they are low-cost relative to the potential cycle time reduction that comes from removing this variability.
4. Run batch tools under a greedy policy
Most wafer fabs have batch operations at which up to eight lots can be processed at one time, where the process time is the same regardless of the number of lots in the batch. Often the process time for the batch is lengthy, as in furnace operations. Because of the long process times, there is an incentive to run these tools with full batches. That way, the capacity for the unused slots on the tool is not wasted. When a batch tool is available and has a partial batch ready to go, there’s a question of whether to go ahead and start the batch or wait for more lots to fill the batch. Some fabs have policies in place to wait for a full (or nearly full) batch.
The problem with this approach is that it drives up cycle time for the lots that are already there (as in the transfer batch case discussed above). Then, after a full batch is run, the batch tool sends highly variable arrivals downstream. The arrival pattern downstream looks like “nothing, nothing, nothing, nothing, … big batch of eight lots”. Often the lots must wait again downstream.
Full batch policies are a particular problem for tools that are not highly utilized – there’s a higher probability of having to wait a long time to fill the batch. There, a greedy policy (just run the tool if there is anything there to run) can generate significantly lower cycle times. An example, generated using simulation, is shown below.
When the tool is highly utilized, it doesn’t matter whether you run a greedy or near-full batch policy, because the batches usually are full (the lines overlap). When you run a greedy policy at those high utilizations, even if you occasionally run a less than full batch, there will be a full batch waiting by the time the partial batch is finished. Batches are usually run close to full.
The greedy policy also works well at low utilizations. The near-full batch policy, on the other hand, is terrible at low utilizations, introducing a considerable amount of unnecessary cycle time. Therefore, we recommend ensuring that your batch tools are being run under a greedy policy, or at least not a full batch. See Issue 9.03 for more details, including a full-fab simulation example.
Impact of Greedy vs. Near-Full Batch Policy on Cycle Time X-Factor for A Tool
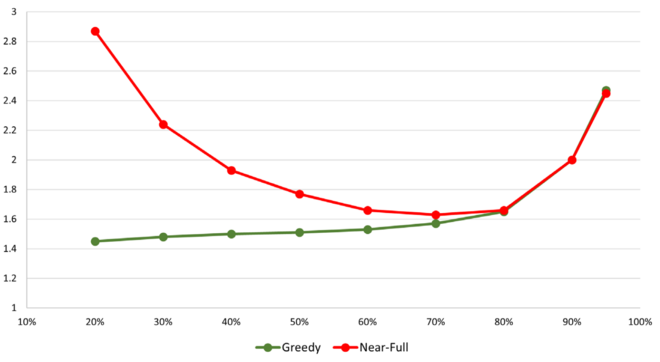
5. Separate maintenance events instead of grouping them
Downtime is a major source of both capacity loss and variability in wafer fabs. Fabs undertake many downtime reduction programs, of course. But one recommendation that we like to make that is not always intuitive for people is to separate maintenance events and other periods of unavailable time.
While it can be tempting to group smaller maintenance activities together, or to group them in with other downtime events, this is generally counterproductive for cycle time. What’s best for cycle time is to have each period of unavailable time be as short as possible, particularly for one-of-a-kind tools, to keep lots moving through the tool smoothly, and prevent WIP bubbles from building up. For cycle time, then, it’s better to break PM activity into the smallest possible chunks and make the tool available for production in between.
Clearly, there are limits to this approach, depending on the qualification time required to bring a tool back up, staffing issues, etc. However, it may be worth checking your PM schedules, to see where you may be introducing more variability into the fab than needed. Tracking average and maximum time offline for scheduled downtime, rather than tracking the time between events, is a very good place to start. See Issue 22.01: On Breaking Up PMs and Other Unavailable Periods, for more detail.
6. Minimize the number of distinct tools for which each operator is responsible, and stagger break schedules
Another often hidden source of cycle time in wafer fabs is operator delay. Any time an operator is responsible for more than one tool, there is a chance that multiple tools will require the operator’s attention at one time. Lot 1 is ready to load on Tool A while Lot 7 is ready to unload from Tool C. Because people can’t be in two places at one time, either Lot 1 or Lot 7 will incur extra cycle time. Tool A or Tool C will also lose some capacity. Operator delay can push a tool to a steeper place on the operating curve, generating a higher-than-expected cycle time per visit.
One way to get a sense of where this is happening is to report what we call Standby-WIP-Waiting Time. Standby-WIP-Waiting is time when a tool is available and WIP is waiting but the tool is not being run. You can’t rely on operators to log a tool into a Standby-WIP-Waiting state, but you can infer it by knowing when WIP has moved out of the previous operation and is now in queue for the current operation. The FabTime reporting module includes Standby-WIP-waiting time on all Tool State-related charts.
Another metric available in some fabs is post-processing time, where the lot has completed processing but not been moved out from the tool. Not all fabs log the end-run transaction as separate from the move out, but those that do can record post-process time. Post-process time is shown on Operation Cycle Time Details charts in FabTime.
Once you know where operator delay may be causing cycle time problems, you can check whether you have operators who are spread too thin, managing too many tools. We’ve had various discussions in the newsletter over the years about whether and how to measure operator utilization. But what we do know is that in the presence of high variability, the more tools you have waiting for a single operator, the more likely you are to incur operator delay.
As a related recommendation, staggering break schedules is best for cycle time (though we imagine that most fabs are doing this already). Dynamic X-Factor is a FabTime metric that can give you a fab-level indictor for where break schedules may be causing trouble.
7. Reduce the number of hot lots in the fab, especially hand-carry lots.
Hot lots are a fact of life in wafer fabs. Hot lots allow a fab to get some small number of lots out with a relatively low cycle time, without having to reduce fab utilization in the way that would be necessary to reduce the overall fab cycle time. But hot lots are not free (despite what some in the organization might believe). Hot lots add variability, which increases cycle time. The greater the number of hot lots you have, the more likely they are to interfere with one another. You can even get into a vicious cycle where you make more lots hot, and the added variability drives up cycle time, leading to a need for even more hot lots.
Front-of-the-line hot lots are less of a problem than hand-carry lots. Front-of-the-line, or regular, hot lots are prioritized ahead of other lots in your dispatch system. However, you typically don’t hold tools idle for them, or break setups. In the best case, front-of-the-line hot lots move queue time from the hot lots to the regular lots, without changing the overall average cycle time. In practice, some capacity is probably still lost at batch tools and tools with setups, which is why we recommend keeping the quantity of regular hot lots below 5% of total WIP.
Hand carry lots (also called rocket lots, platinum lots, and many other names) are more disruptive. Any time you hold tools idle ahead of a lot that is expected, you lose capacity. Similarly for breaking setups, running batch tools nearly empty when full batches of other lots could have been run, etc. Hand carry lots also typically require extra management attention, which is thus diverted from other activities. Our recommendation, if you must have hand-carry lots, is to have no more than one or two in the fab at the same time.
8. Smooth the flow of arrivals into the fab
At the tool level, arrival variability can be a significant contributor to cycle time. Performance is much better under a steady pattern of arrivals than a lumpy pattern of arrivals. Arrival variability is less of an issue for major bottlenecks that always have a large queue waiting but can degrade cycle time performance quite a bit for tools with moderate utilization. The discussions above about batch transfer and batch processing were centered in part around minimizing arrival variability throughout the process flow. A source of arrival variability that is more directly controllable is lot releases into the fab. For cycle time, it’s generally better to release fewer lots at a time steadily throughout the day, rather than releasing a large batch of lots into the fab at one time.
While the arrival variability from lumpy lot release policies does dampen as WIP makes its way through the process, variability from lot release can have a negative impact on cycle time at operations early in the process flow. An example from the FabTime demo model is shown. The coefficient of variability of arrivals is shown to each operation, in operation order. This data is noisy (reflecting sources of variability such as batch tools) but shows a clear trend downward over the set of operations included.
Some fabs do release lots in batches specifically timed to optimize loading of early batch tools. This makes sense if those batch tools are highly utilized. But, as discussed above, this strategy may be introducing unnecessary variability in cases where the batch tools are more lightly loaded overall.
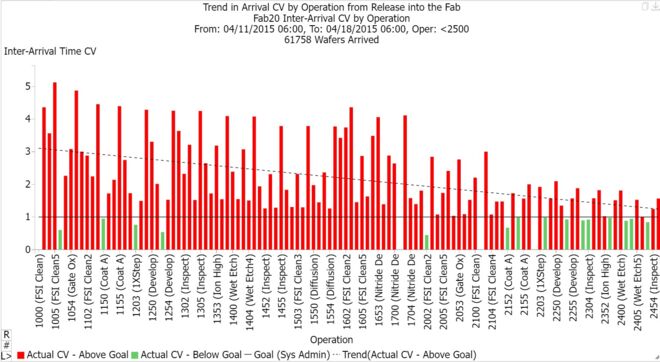
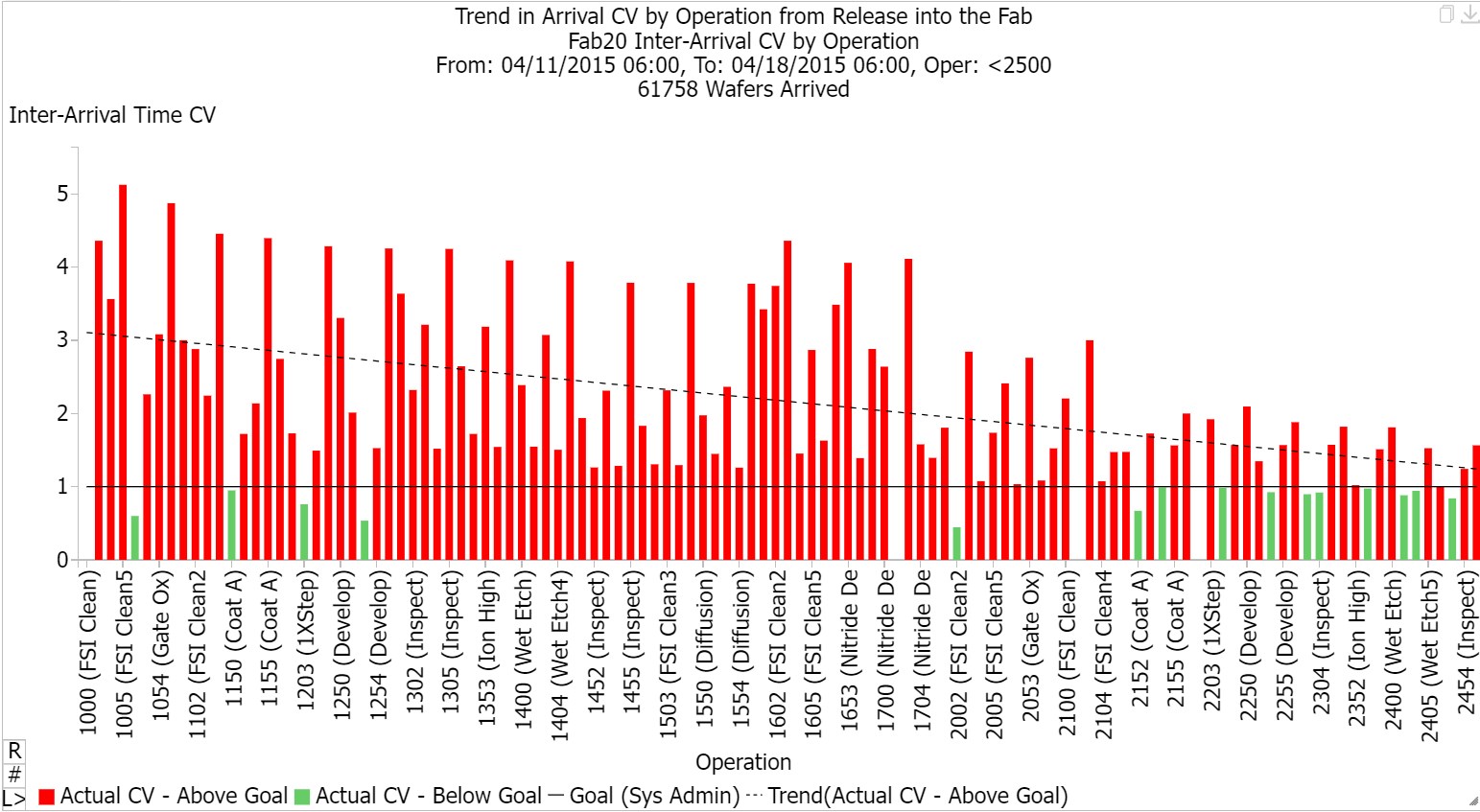
9. Check setup avoidance policies to make sure that low volume lots aren't waiting too long, especially on non-bottlenecks
Some tools, such as implanters, require significant setups when changed over from running one type of process to another. Setup avoidance policies are dispatch policies that attempt to minimize that setup time. In the simplest implementation, a setup avoidance policy will prioritize lots of the same recipe over other lots. If there is a lot in queue that doesn’t require a setup, that lot will be processed. The setup will only take place if there are no lots of the current recipe waiting. In some cases, operators may even go further, waiting until other lots of the same recipe arrive, so as not to incur the setup time.
Setup avoidance policies are important in minimizing lost capacity on key tools. Setup time is non-value added. Operators who are measured based on moves have an incentive to do as few setups as possible. However, what can happen in the presence of setup avoidance policies is that lots from low volume routes can wait … forever.
We recommend having some threshold in place by which, if a lot waits more than some amount of time, a setup is performed, even if there are lots with the current recipe in queue. This is especially important for non-bottlenecks, where the loss of capacity from doing the setup isn’t a major problem anyway. We also recommend having policies in place that discourage waiting for a lot with a matching recipe when there are lots already there that could be processed (after a setup). This is analogous to the greedy vs. full batch decision.
10. Make dispatching decisions to keep critical downstream tools from starving
This recommendation is last on our list not because it is not important, but because it requires more effort to implement. Dispatch decisions (which lot to process next on each tool) are normally local, as compared to scheduling solutions, which attempt to optimize across the entire fab. A useful first step in making dispatch decisions a bit more global is to incorporate downstream information into the decision with the goal of keeping critical downstream tools from starving, and balancing WIP across the fab.
WIP smoothing or line balance policies look some number of steps downstream for each lot in queue and prioritize lots that have the least WIP ahead of them. We have done some research that showed that in the presence of extended downtimes, a Critical Ratio (CR) dispatch rule (common in fabs) can lead to undesirable behavior – WIP bubbles that oscillate between the front and back of the line.
If you can instead focus on keeping your line balanced (roughly equal WIP throughout segments or sub-segments), you may find your fab easier to manage. You’ll see fewer WIP bubbles, fewer starved bottlenecks, and lower cycle time variability. This lower cycle time variability will end up helping with on-time delivery. You can also still use CR as a secondary dispatch factor, of course, but we recommend that line balance be a primary goal of your dispatch policies.
In general, once you have tackled the low-hanging fruit from recommendations 1 through 9, a logical next step is to move from local dispatch rules to rules that incorporate downstream information into upstream decisions. The next step after that is to move to an advanced scheduler, such as the INFICON Factory Scheduler.
Conclusions
Fabs are usually struggling with competing pressure to reduce cycle time and increase tool utilization. In the current chip shortage, these pressures have been exacerbated. While there are efforts underway around the globe to add wafer fab capacity, these initiatives (particularly those involving new fab construction) take time.
The good news is that it is possible to reduce fab cycle time by reducing variability. When successful, variability reduction gives fabs a choice. They can achieve better cycle time, or they can push to a slightly steeper place on the operating curve and squeeze out a bit of extra throughput. These days, even a small improvement in either would be welcomed by most fabs.
In this article, we have shared ten recommendations for improving fab cycle time through changes in operating practices. Most of these recommendations are relatively low cost, with the possible exception of recommendation 10 (modifying dispatch rules to include downstream information). They rest on understanding the way that decisions made by people in the fab, from lot release to PM scheduling, affect variability.
Most of these recommendations will not be surprising to people who understand fabs. But we hope that they will serve as a reminder, or possibly as ammunition to help spur changes. We welcome your questions and feedback.
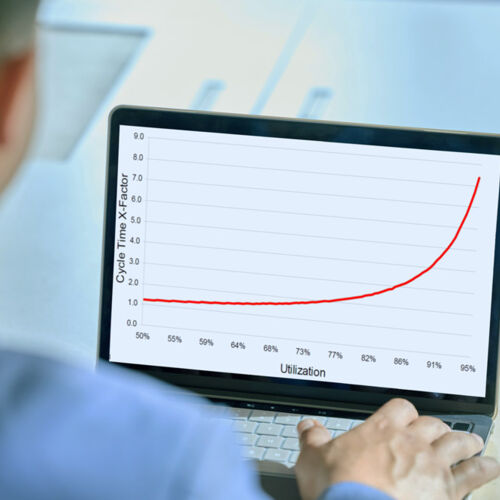