A Fab Cycle Time Improvement Framework
How to estimate the potential cycle time improvement from different strategies to determine what will have the greatest benefit.
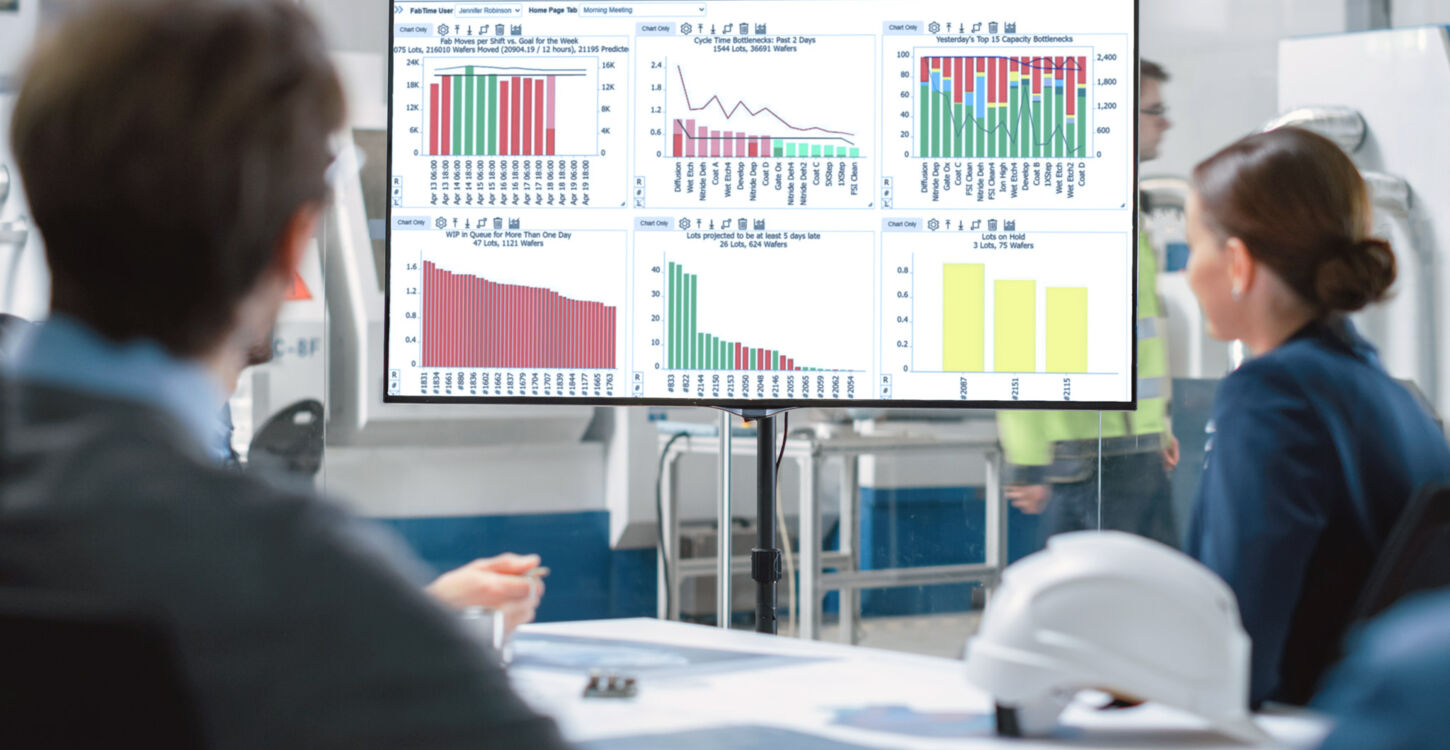
There are various ways to decide which specific tools to focus on for cycle time improvement, but how do you decide which initiatives matter the most for your fab? The truth is that fabs are extremely complex systems containing many sources of cycle time. For the most part, it’s not possible to predict the expected cycle time improvement from any single approach in isolation. However, in thinking about this question, we realized that we do have a sense, after working with fabs for many years, of a logical order in which to introduce improvement initiatives. In this article, we discuss the contributors to fab cycle time and propose a framework for implementing cycle time improvement recommendations for your fab.
Contributors to Fab Cycle Time
There are three fundamental conditions that increase cycle time in a fab (or any manufacturing system). They are:
- High utilization of equipment (having a low buffer of standby time).
- Variability in arrivals to tools and/or in lot-to-lot process times at tools.
- An insufficient number of tools for a given operation, due to either small or one-of-a-kind tool groups or to process restrictions/constraints.
There are many factors in wafer fabs that contribute to cycle time. These include:
- Downtime (scheduled and unscheduled)
- Product mix
- Holds
- Setups
- Time constraints between process steps
- Lot release
- Operator unavailability
- Scheduling/dispatching
- Engineering use of tools
- Running development lots in a production fab
- Hot lots
- Reentrant flow
- Scrap
- Rework
- Manual lot transport between steps (via carts or runners)
- Automated material handling systems
- Batch processing
- Soft dedication/operator preferences for tools
All these complexities affect cycle time via their impact on one or more of the fundamentals. For example, downtime reduces buffer capacity on tools, driving up utilization. Downtimes contribute to variability and temporarily reduce the number of available tools per tool group. Setups take up capacity on tools, also reducing buffer capacity. They also effectively increase the process time of the lot at the front of the queue when a setup occurs, increasing effective process time variability.
It is difficult to quantify the impact of any one of these factors on overall cycle time, because fabs are so complex and so different from one another. For a small, high-mix fab, hold reduction could be important. For an operator-constrained fab, operator unavailability might be more significant. Further, many of these factors interact with one another, making decisions about improvement programs challenging. For example, process restrictions may well improve yields, cutting back on the process time variability that comes from different lot sizes. But process restrictions that lead to single or dual path operations increase cycle time.
That said, there are things we know from working with fabs over many years:
- When asked about the top contributor to their fab’s cycle time, more people mention downtime than anything else.
- Single path operations are not a problem for everyone, but where they occur, they increase cycle time significantly.
- Product mix and holds have increased in complexity and prevalence over the years.
- Delays due to lack of operators can cause forced idle time, reducing buffer capacity and driving up cycle time.
- Many fabs have worked successfully over the years to reduce the quantity of hot lots. Hold reduction efforts don’t appear to have progressed as far.
These observations inform our framework for tackling wafer fab cycle time improvement project, proposed below.
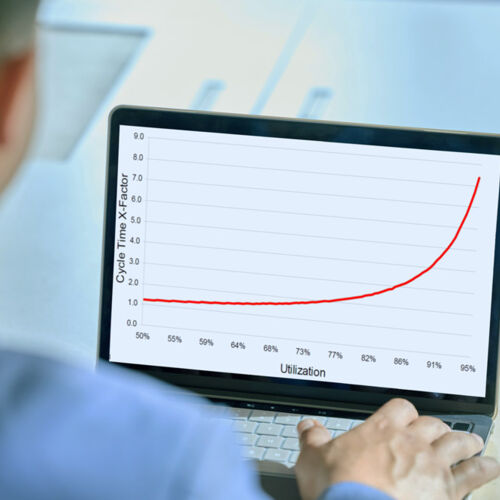
Free FabTime Operating Curve Generator
A Proposed Cycle Time Improvement Framework
In the conclusion to our cycle time class, we group our top recommendations according to the three fundamentals. That grouping is helpful in summarizing the material from the course. However, as our subscriber question above demonstrates, this still doesn’t tell a specific fab where to start and where to focus. Here, we attempt to rectify that omission. We welcome your feedback.
Step 1: Focus on Number of Qualified Tools
If your fab has any operations that have only one or two qualified tools (that are not one-of-a-kind or two-of-a-kind tools), the highest “bang for the buck” thing that you can do to improve cycle time is work to change that. For every operation where you can get from one qualified tool to two, you’ll reduce that operation’s cycle time by about 50%. For every operation where you can get from two to three, you’ll likely reduce that operation’s cycle time by another 20% to 30%. The benefit diminishes after that, as shown below. (See more detail in Issue 20.05: The Impact of Tool Qualification on Cycle Time.)
Once you are in a situation where you either have no more single or dual path operations, or only have single or dual path operations on tools that are true one-of-a-kind or two-of-a-kind tools (see Issue 23.05: Managing One-of-a-Kind Tools for suggestions here), move on to Step 2.
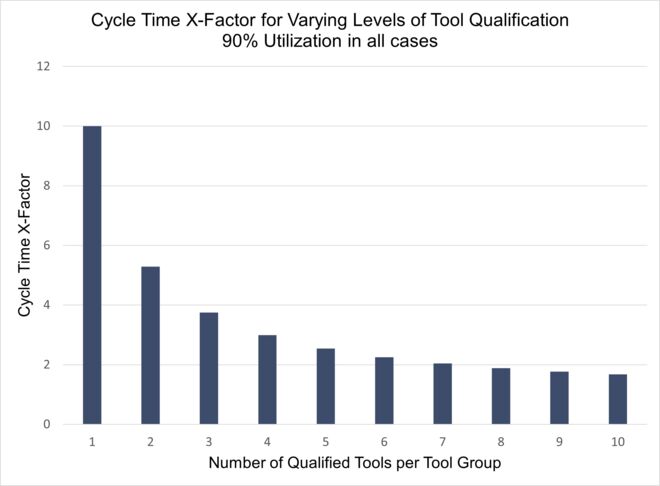
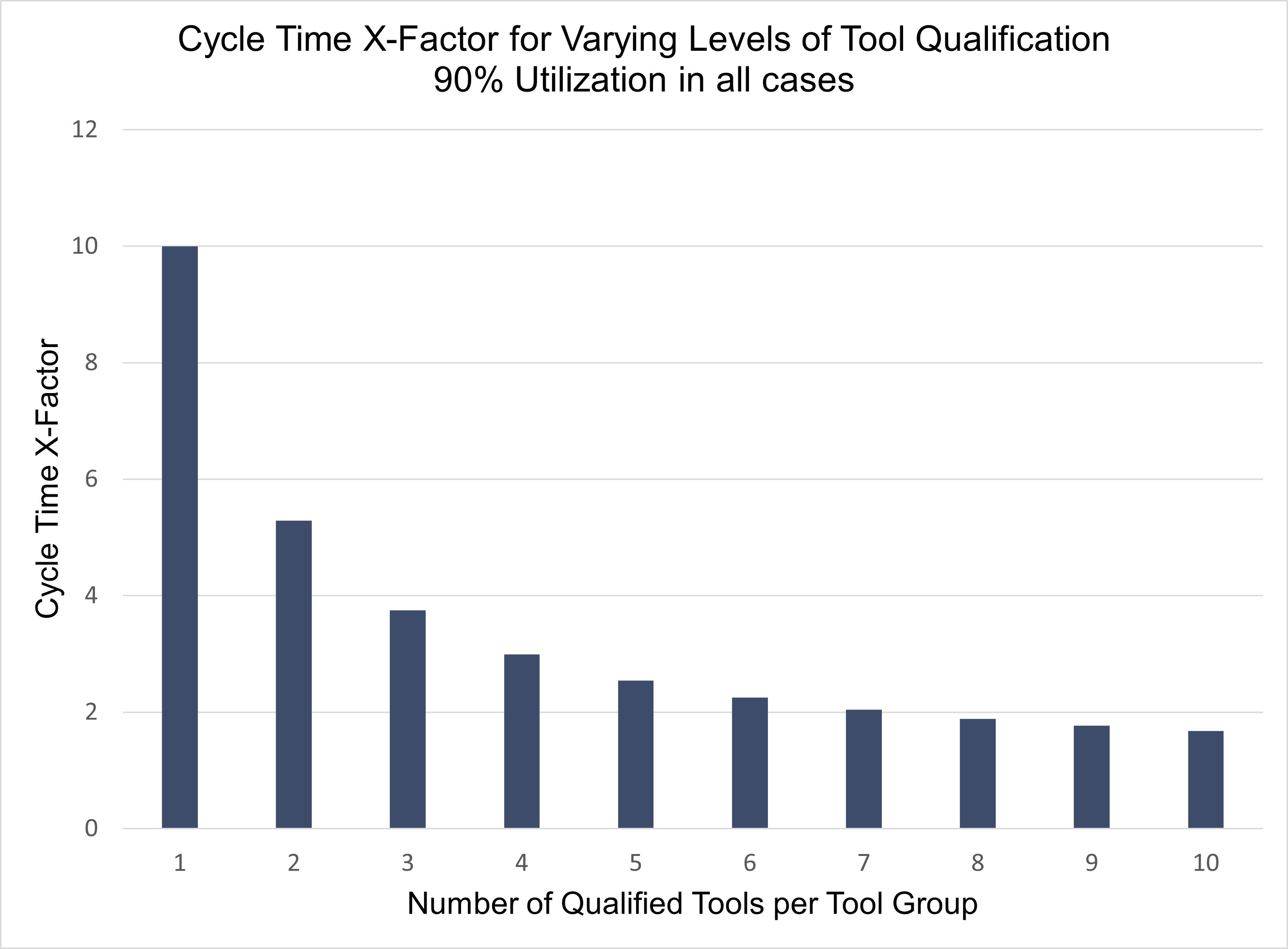
Step 2: Find the Current Cycle Time Bottlenecks
Measure the operation level cycle times by tool group (over at least two weeks) and look for tool groups that have a high per-visit X-Factor (or just a large amount of queue time). In the example below, Develop, 5xStep, Nitride Deh2 and Nitride Deh are all cycle time bottlenecks. Also consider using the WIP Hours metric (a metric from the FabTime module that shows the total amount of process time required for all WIP in queue for each tool group). Identify a candidate list of up to 10 tool groups that right now are driving up your fab’s cycle time.
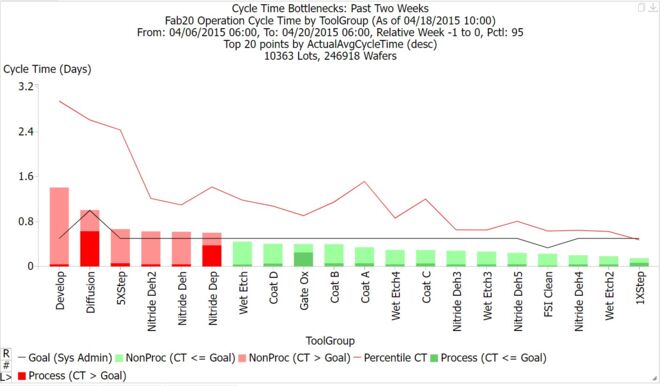
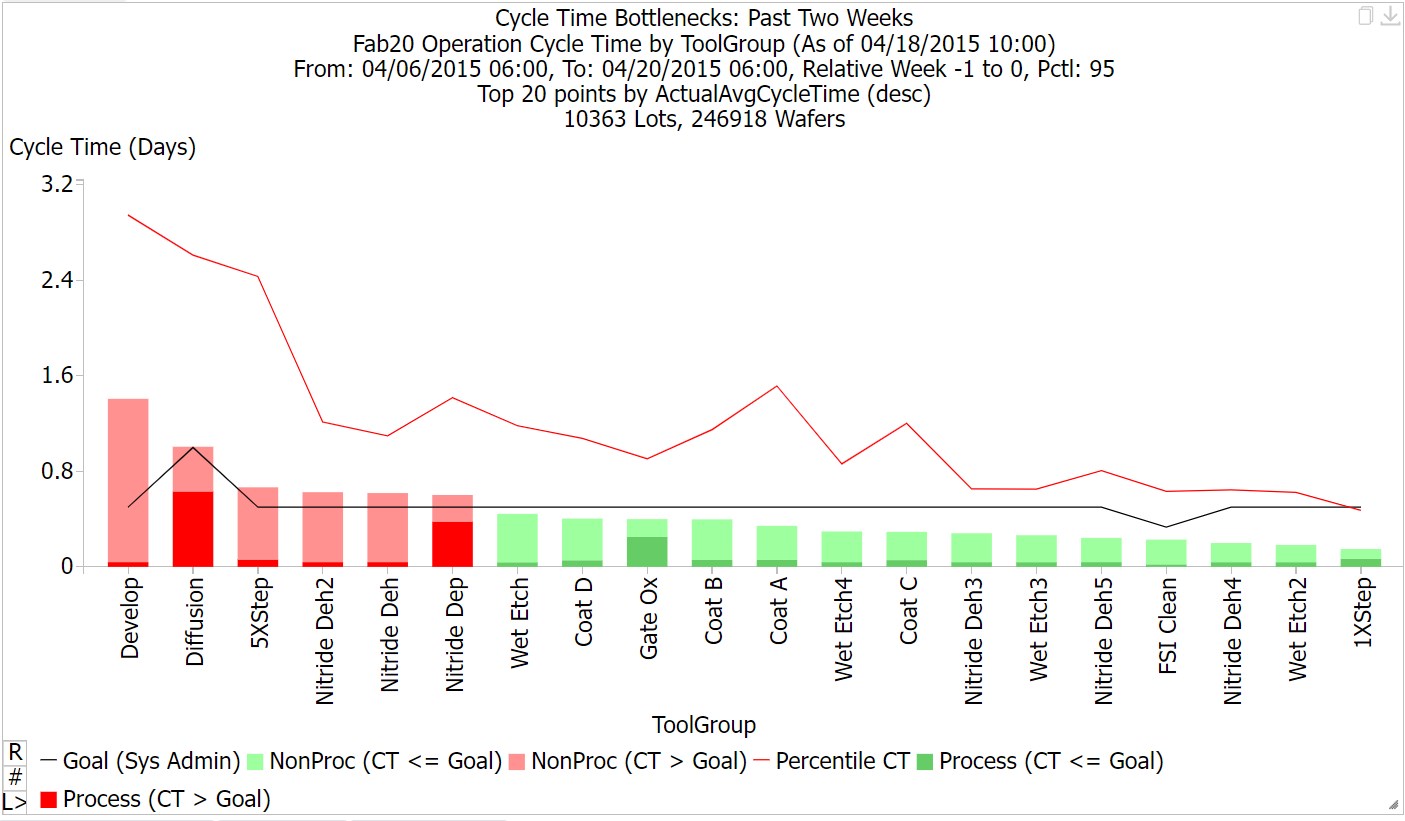
Step 3: Analyze the Cycle Time Bottlenecks to Understand Root Causes
For each of the cycle time bottlenecks, the next step is to determine the likely root causes. This will help to inform which of Steps 4 through 7 are likely to be of most benefit for your fab. Here are a few avenues to explore.
- Is there a very low amount of standby time? Make sure you’re looking at true standby time, not standby time when there is WIP waiting, because that’s more of an operator delay. If you have a tool group that has a high cycle time per visit, and a low amount of true standby time (5% or less), focus on increasing buffer capacity for this tool group, as outlined in Step 4.
- Measure Green-to-Green (G2G) time if possible. G2G is a metric that captures the total time that a tool is unavailable from when it first goes down until it comes back up, grouping together sub-states such as PM and unscheduled downtime. Look for the tool groups that have long periods of unavailable time (whether from scheduled or unscheduled downtime events). Look especially at tool groups with long downtime that are smaller tool groups. This is where downtime variability is likely to be causing the most problems. These are tool groups where you should focus on reducing downtime variability, as discussed in Step 5.
- Is there a high amount of arrival variability? Ideally you would look at the coefficient of variation of the time between arrivals to each tool group of interest (see example below from the FabTime demo server). However, a proxy for this is to look at a graph of arrivals per shift and see where that is highly variable. See if you can dig into the data to understand why the arrival variability is high at key tool groups. Are they fed by a large batch tool? Are they downstream from a tool with a lot of downtime variability? Are carts being used for manual transfer of lots to this tool? Once you have an idea about the sources of the arrival variability, move on to Step 6.
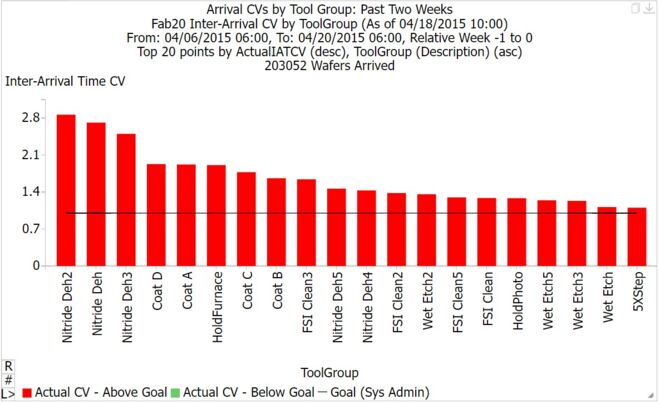
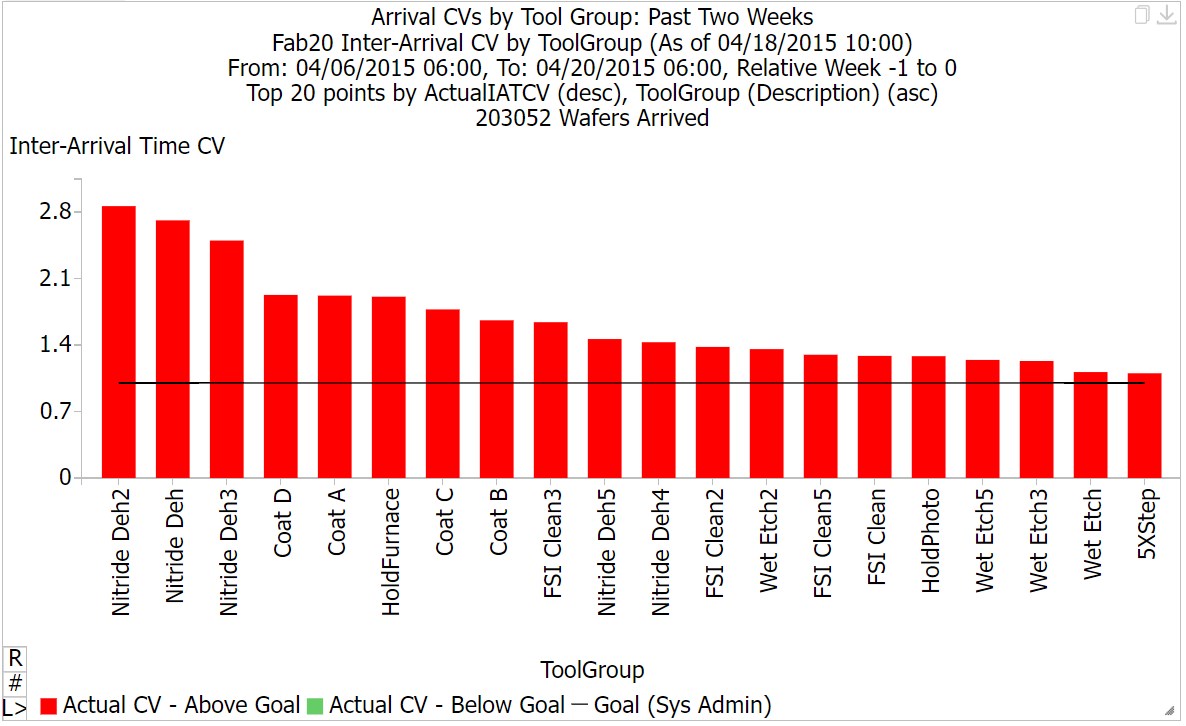
Step 4: Increase Buffer Capacity at Key Tools
Because of variability in the fab, it’s important for all tool groups to have some standby time. This standby time provides a buffer against variability and keeps cycle time from getting too high. If you have tool groups with little or no standby time, they are likely cycle time bottlenecks. Recommendations for improvement here include:
- Maximize availability. These are the tools for which it is most important to focus downtime reduction efforts. Any unavailable time that can be converted to standby time results in a lower effective utilization (for the same amount of process time) and reduces cycle time.
- Minimize forced idle time. Forced idle time is time when the tool is available and has WIP that could be run, but for some reason is not running. Often, though not always, forced idle time results from operator unavailability. Standby-WIP-waiting time (a category that we report on the FabTime Module Tool State Trend and Pareto charts) is a useful indicator of forced idle time. Post-process time (time between when a tool logs an End Run event and an operator logs a Move Out from the tool) also indicates a hidden forced idle time. Here, the lost capacity will be hidden in inflated process times. In addition to operator unavailability, forced idle time can result from hand carry lots (the tool is held idle to wait for an arriving hand carry) and from time constraints between process steps (hold the lot at the upstream tool until we are sure it can make it through the downstream step). Dispatch decisions that starve downstream bottleneck tools also effectively force idle time. Suggestions for reducing forced idle time include:
- Minimize the number of distinct tools for which each operator is responsible, and stagger break schedules.
- Reduce the number of hot lots in the fab, especially hand-carry lots that cause setup changes or force idle capacity.
- Make dispatching decisions to keep critical downstream tools from starving.
- Make sure that lots aren’t sitting on hold unnecessarily when they could be in process. For example, make sure that a backup is designated for future holds, in case the original engineer is unavailable when the lot reaches the future hold step. This can create a type of forced idle time, if a tool could have been busy processing the lot.
- Transfer processes to other tool groups. Some fabs that have significant tool redundancy have different tool groups that contain similar tools. A larger tool group may be broken into two smaller groups. This can make sense provided the tool groups aren’t too small, but it can also lead to unbalanced utilizations between the sub-groups, particularly if product mix changes. In such cases, it may be necessary to transfer operations between sub-groups. It is always a good idea in these situations (especially for high mix fabs) to keep a close eye on the utilization of each sub-group and make changes as necessary.
- Find and reduce soft dedication. Soft dedication is common in fabs. It occurs when the capacity model and tool qualification data in the MES say that a tool group is fully cross-qualified, but the operators in fact have preferences for certain tools over others. This can result in unbalanced utilizations across a tool group, where one tool is used more than expected, and others less. To identify soft dedication, plot the moves and availability efficiency of each tool in the group, and look for mismatches. Where this is taking place, the operators doubtless have reasons (one tool is in a less convenient location, a newer tool is faster than the other tools in the group, etc.). However, if soft dedication is causing cycle time bottlenecks, intervention on the part of management may be required.
Step 5: Improve Variability from Downtime
As mentioned above, downtime is recognized as one of the largest sources of cycle time in most fabs. Improving overall availability increases buffer capacity, as discussed in Step 4, and is a long-time focus of fab improvement efforts. What is sometimes less well-understood is the impact of downtime variability on cycle time. To improve cycle time, it’s best to minimize that variability. To do this:
- Separate maintenance events instead of grouping them.
- Focus downtime improvement programs on reducing the duration of repair times, particularly for the cycle time bottlenecks. This might mean purchasing additional spare parts or re-allocating equipment technicians where long total unavailable times are driving high cycle time. Reducing the duration of downtimes will have the highest impact at one-of-a-kind tools.
- Report not just availability but availability variability. Use the Average vs. Coefficient of Variation (CV) of Availability chart in FabTime, or use A20/A80. Look at hours of unscheduled downtime broken up by sub-state, to understand how much time is spent waiting for parts, waiting for technicians, etc., to help identify the best paths to improvement.
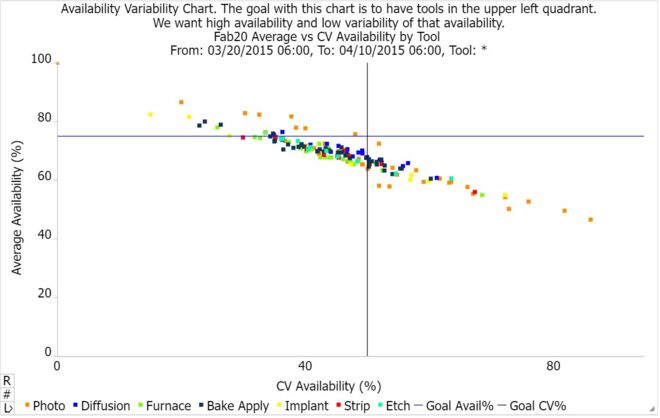
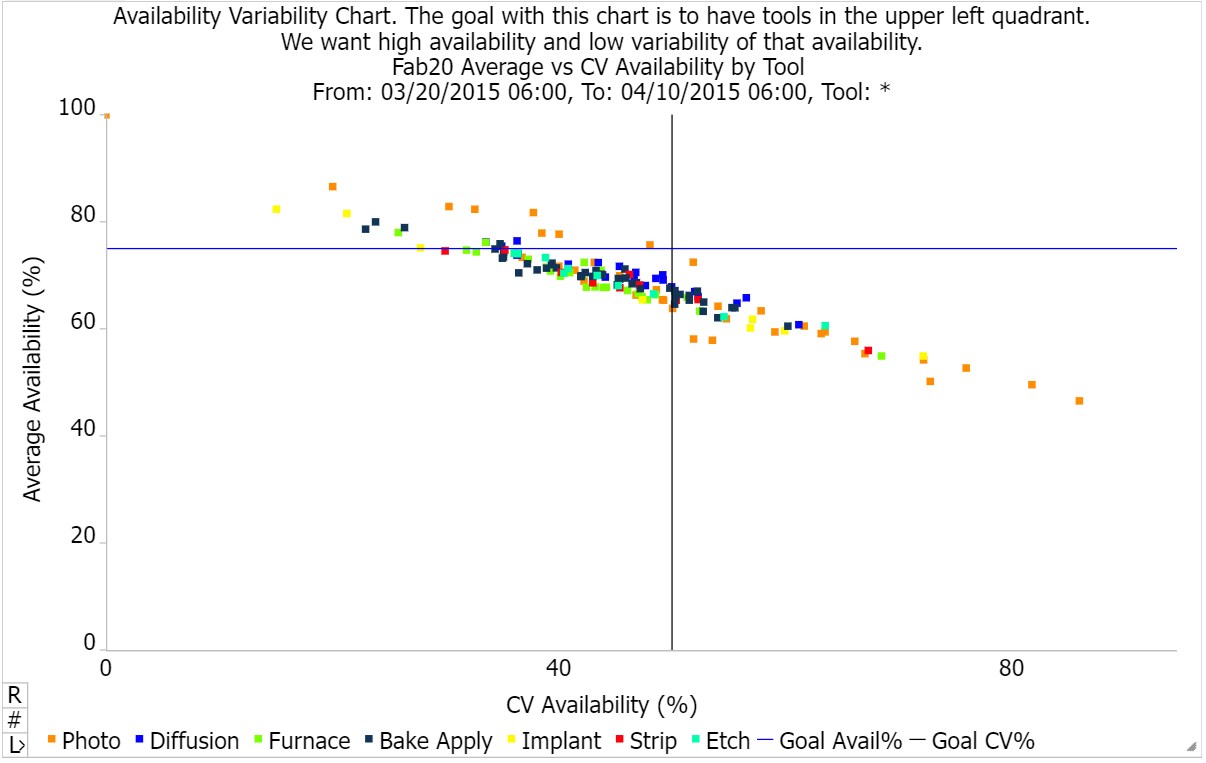
Step 6: Improve Arrival Variability
Variability changes the shape of the operating curve of cycle time x-factor vs. utilization. Reducing arrival or process time variability reduces cycle time. In the queueing formulas that we teach in our cycle time class, arrival variability and process time variability, if equal, have the same impact on cycle time. Usually, however, there is more arrival variability than process time variability in a fab. This is because process time variability often gets sent downstream as arrival variability to the next step. Meanwhile, other aspects of semiconductor manufacturing contribute additional arrival variability. Efforts to reduce arrival variability should focus on the following areas.
- Batch processing: Batch tools, such as furnaces, are tools that can process multiple lots at one time. The arrival pattern downstream from a batch tool consists of long periods of no arrivals, and then a burst of several arrivals at once. This variable arrival pattern contributes to high cycle times downstream from batch tools. Batch process time is usually independent of the number of lots in the batch. This leads to a decision when a partial batch is available for processing, but not a full batch. Some fabs have policies that require waiting for some minimum batch size before processing. This is fine for tools that are heavily loaded, because most batches on these tools end up full or nearly full anyway. However, requiring full batches on less heavily loaded tools increases queue time for the lots that must wait and sends extra arrival variability downstream. We recommend working to eliminate minimum batch size constraints on batch tools that are not heavily loaded.
- Lot transfer between steps: A similar issue to batch processing arises when carts are used to transfer lots between steps. Operators, in an understandable effort to increase their own efficiency, often wait until a cart is full before moving it. This means that lots wait on the cart, and then wait again when sent downstream, as they wait for other lots on the cart. Due to operator constraints, it may be necessary to use carts in this way. However, we note that one possibility for reducing arrival variability in the fab is to get smaller carts for lot transfer or set policies that discourage waiting until a cart is full before moving it.
- Lot release into the fab: Lots are typically released into the fab in batches (groups of lots). The less frequent those lot releases are, the more variable the arrival pattern to the early operations in the process flow. If you find that arrival variability is high at early steps in the flow, consider more frequent, smaller lot releases.
Step 7: Improve Process Time Variability
While not usually as pronounced as arrival variability, process time variability can also be considerable in fabs, especially high mix fabs. The simplest type of process time variability involves running recipes with different process times on the same tool. Things like downtime and setups also contribute to effective process time variability. From the perspective of a lot at the front of the queue and ready to go, a downtime or a setup looks like an increase in process time. Operator unavailability to unload tools also contributes to process time variability, as do changes in lot size due to scrap and rework. Steps to help reduce process time variability include:
- Work on process simplification. The more similar your recipes (and process times) are, the better for cycle time. There are other benefits from this approach, too, in terms of learning curves and a more robust response to product mix changes.
- Reduce setups and rework. One thing to be careful of when reducing setups, however, is to check setup avoidance policies to make sure that low volume lots aren’t waiting too long, especially on non-bottlenecks. We recommend having some threshold in place by which, if a lot waits more than some amount of time, a setup is performed, even if there are lots with the current recipe in queue. This is especially important for non-bottlenecks, where the loss of capacity from doing the setup isn’t a major problem anyway. We also recommend having policies in place that discourage waiting for a lot with a matching recipe when there are lots already there that could be processed (after a setup). This is analogous to the greedy vs. full batch decision and introduces some forced idle time.
- Consider breaking up large tool groups into smaller groups and assign recipes with similar process times (and/or that reduce setups). This must be done with caution, however. The tool groups shouldn’t be smaller than 3-4 tools, and it’s important to balance utilization across such sub-groups, as discussed above.
- Automate reporting of End Run transactions so that it’s possible to separate post-process time from true process time. Where this is significant, look at assigning/cross training more operators.
Step 8: Consider Other Fab-Wide Initiatives
One shortcoming of the approach described here is that by focusing on specific cycle time contributors, we may be under-emphasizing fab-wide initiatives, including:
- Reduce holds, especially future holds. This will reduce variability and help reduce the cycle time of individual lots.
- Enhance dispatch rules to take into account non-local information (to spread out WIP, avoid feeding WIP to down tools, etc.).
- Implement Smart Scheduling technology, such as the INFICON Factory Scheduler.
Conclusions
Fabs are uniquely complex environments, with no shortage of factors that increase cycle time. We have listed some of these factors above, though we have doubtless missed a few. This complexity can make it challenging to even know where to start with improvement programs. For this article, we have taken a step back to think about how the various recommendations that we have made over our years in the industry could fit together to provide a logical sequence for cycle time improvement efforts.
We stand by our top recommendation for improving fab cycle time, increasing the number of qualified tools for each operation, as a first step. After that, we think it makes sense to identify the tools that are contributing the most to fab cycle time, and then analyze them to get a sense of likely root causes. After that, we would focus first on increasing buffer capacity for these cycle time bottlenecks, then on reducing variability from downtime, then on arrival variability, and then on process time variability.
We have included other fab-wide efforts such as hold reduction and enhancing dispatch rules as a final step in the framework, through these projects could be undertaken at any point. Of course, this sequence, and the recommendations therein, will not be a perfect fit for every fab. But we believe that many fabs could benefit from thinking about cycle time improvement in this sequential way.
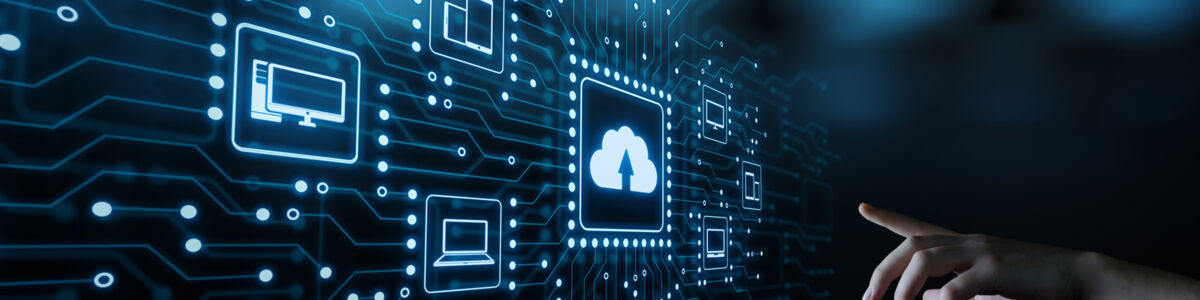